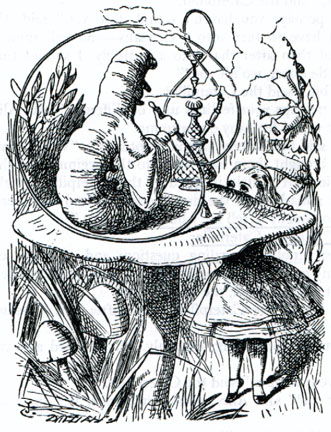
Like the Internet of Things that shed its drab M2M image to become the centerpiece of the digital transformation of industrial enterprises, artificial intelligence is sprouting a new life from its 50-plus years old roots. (Yes, we’ve been doing AI, admittedly with limited success, since the late 1950s.)
Conversations about AI seem to follow a course similar to that of the IoT narrative. Initially, IoT pundits were obsessed with the ability to connect billions of “things” to the Internet. Not only did most of these predictions proved overly optimistic, but the connection between sheer connectivity and meaningful business outcomes was loose, at best.
Today’s IoT narrative shifted to focus on business outcomes enabled by the data generated by connected devices. Industry matured from counting conduits to measuring the value of their content. Read More