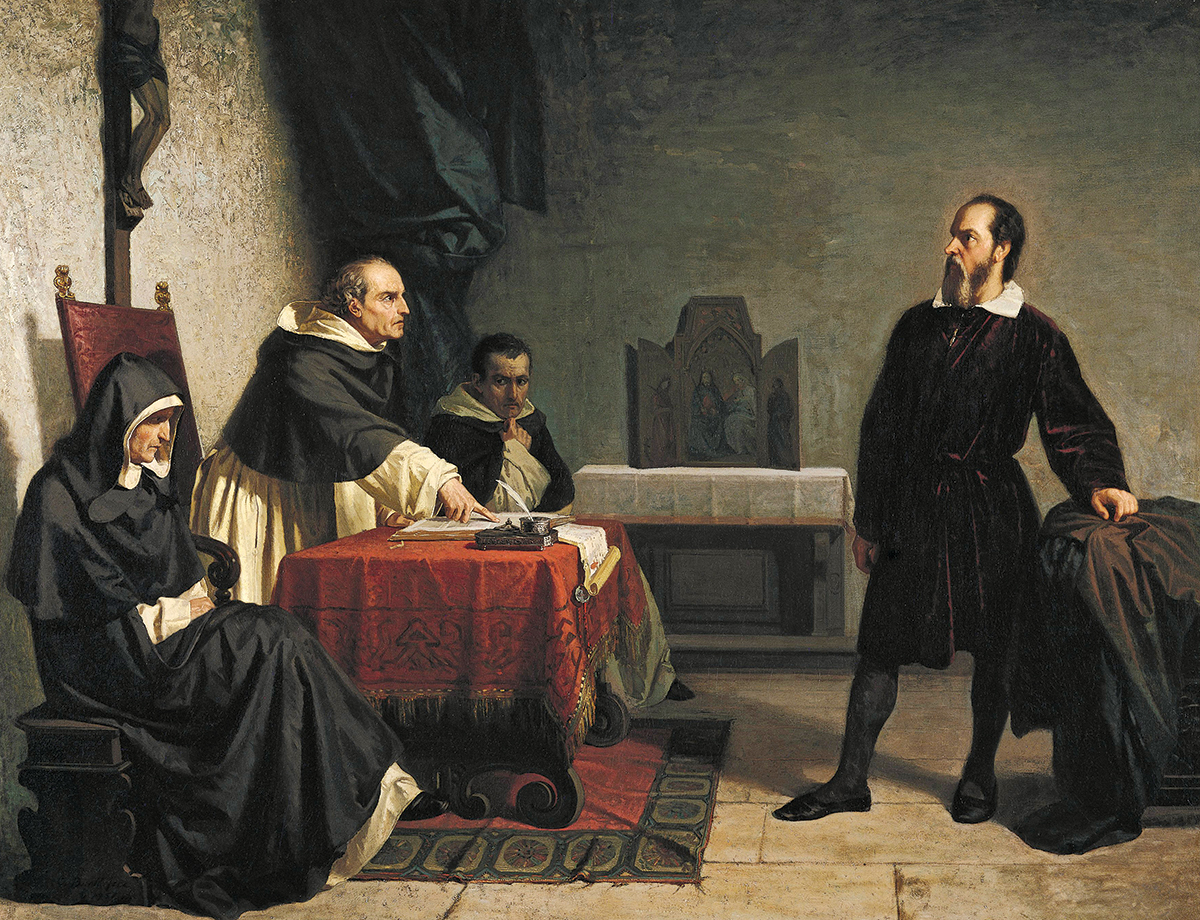
Much of the buzz about the industrial Internet of Things (IoT) and predictive algorithms is calling service organizations to adopt predictive maintenance (PdM) methods and tools. In fact, PdM is one of the frustratingly few-well flashed-out use cases for IoT.
The rationale for adopting predictive maintenance is quite convincing.
Many service organizations schedule equipment maintenance activities based on statistical models. Routine preventive maintenance (PM) schedule is based on average failure rates of components and systems and prescribes mandatory part replacement and other maintenance activities before a critical failure is likely to occur.
While generally effective, PM is fundamentally inefficient and wasteful. Because maintenance activities are scheduled well ahead of the anticipated end of life of components, systems are taken out of service prematurely, parts are replaced while they still have many hours of service left in them, and labor hours are wasted unnecessarily. In mission-critical systems such as airplanes to elevators, where PM schedule is very aggressive and errs on the side of safety, PM is a costly activity.
Condition-based maintenance (CBM) is potentially a more economical approach because it calls for remedial maintenance activities based on objective evidence of need rather than on a rigid predefined schedule. However, not all failure modes can be monitored and detected cost-effectively, and frequently the time window these monitoring methods provide is too short, resulting in an unscheduled downtime for repair anyway.
Predictive maintenance systems collect rich machine health information from instrumented assets and use sophisticated predictive algorithms that anticipate failure much earlier than CBM. The promise of PdM is to issue an early warning with enough lead time to schedule a remedial action at a time that has the least negative impact on the business.
Is Predictive Maintenance Counterproductive?
Despite the sound business logic of PdM, some service organizations and maintenance experts make passionate arguments against PdM. Predictive maintenance practices, they say, are disruptive and counterproductive.
Large industrial plants employ rigid maintenance schedules that prescribe periodic preventive maintenance activities in regular intervals. These scheduled activities are performed at precalculated frequency and intensity such that unscheduled disruptions are sufficiently infrequent. And as the cost of these planned shutdowns is baked into the routine operation model of the maintenance organization, it tends to go unnoticed.
In these organization, adherence to PM is paramount, and an out of schedule maintenance activity is disruptive. Moreover, unscheduled maintenance event have little, if any, influence upcoming scheduled maintenance that now introduce redundancy and waste. Under this regime, PdM can be easily construed as unnecessary and disruptive and the balance is often tipped in favor of the tried-and-true PM schedule.
To assess this argument, let’s look at the possible scenarios of a PdM software issuing an alert about an imminent failure:
Scenario One: The PdM software predicts a failure will occur before the next scheduled maintenance activity
This is great! The system just prevented an unexpected downtime that would have occurred before the next scheduled PM. With an early warning and detailed failure information provided by the system, the maintenance organization has ample time to decide when to perform the necessary repair. Moreover, the maintenance technician can take the opportunity to perform other routine tasks and possibly defer some or all of the next PM activities, thereby improving the asset’s uptime.
The potential savings are significant, and because the fundamentals of preventive maintenance, such as failure modes and mean time between failures statistics, still govern the overall schedule, no additional risk is involved.
Scenario Two: The PdM software predicts a failure will occur later than the next scheduled maintenance activity
Great, no loss here, and the next scheduled maintenance will be more precise and effective, especially if the predicted failure mode isn’t easily discernable during routine PM.
Scenario Three: The PdM software predicts a failure incorrectly (false positive)
Here you will incur operational loss because you service the equipment sooner than is needed. Nevertheless, you can use the opportunity perform some routine inspections and preventive activities that would have been done during the next PM, and push some activities for later.
A good PdM software should exhibit a low rate of false positives and the waste introduced by these infrequent events should be significantly less than the waste built into a normal PM schedule. However, admittedly, false positives could create a negative impression whereas the waste in PDM is built into the operations and is invisible.
Scenario Four: The PdM system did not report an impending failure (false negative)
Well, this is no different than the PM practices of today.
Rethink the Approach and Rerun the Numbers
Maintenance organizations and regulators have been conditioned to believe that the only way to achieve their objectives for reliability and safety is by adhering to strictly scheduled maintenance procedures.
Continued fast advances in IoT instrumentation and connectivity that feed data into sophisticated analytic and predictive algorithms give maintenance organizations and regulatory bodies a strong foundation to look again at PdM. The right mix of PM and PdM methods will minimize unnecessary preventive maintenance tasks and reduce operations and maintenance expenditures while maintaining and possibly improving the mandated reliability and safety levels.