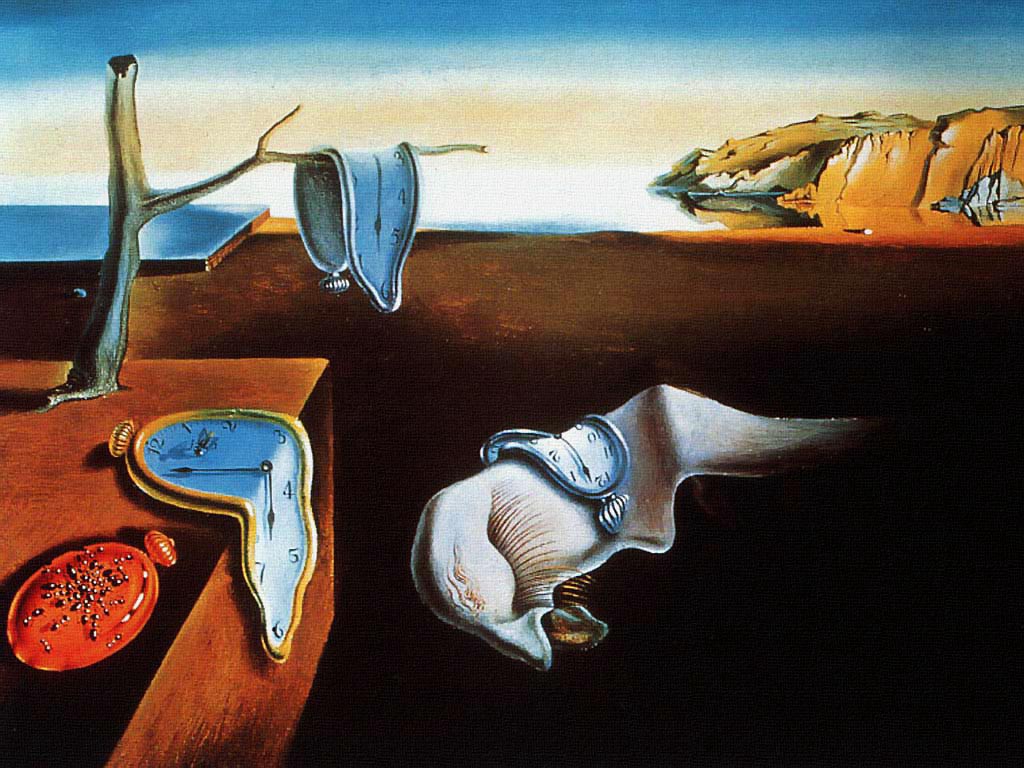
Many tasks in which humans excel are extremely difficult for robots and computers to perform. Especially challenging are decision-making tasks that are non-deterministic and, to use human terms, are based on experience and intuition rather than on predetermined algorithmic response. A good example of a task that is difficult to formalize and encode using procedural programming is image recognition and classification. For instance, teaching a computer to recognize that the animal in a picture is a cat is practically impossible to accomplish using traditional programming.
Artificial intelligence (AI) and, in particular, machine learning technologies, which date back to the 1950s, use a different approach. Machine learning algorithms analyze and classify thousands of images of cats and an equally large number of images of backgrounds that do not contain cats, and “learn” how to recognize cats even in pictures they have never analyzed before. In other words, machine learning algorithms are inductive: they use data to generalize from instances to behavior, and respond to future inputs that are not necessarily identical to past examples. Obviously, these algorithms can only recognize objects they have learned.
Enthusiastic headlines and bold promises about AI make machine-learning technologies seem nothing short of magic, and suggest they provide an easy answer to almost any complex decision-making task we wish to automate.
But effective and reliable machine learning is difficult, and these systems often fail to deliver on the promise. The data used to train these systems may not have sufficient range and variety of patterns, and may include invalid and misleading examples. Furthermore, there’s no easy proof or measurement method to determine whether the training data is complete or even sufficient. Worse, training datasets of human decisions are very likely to include cultural, educational, gender, race and other biases, whether intentional or not.
Setting self-learning algorithms free to search for new information to enrich their knowledge about the world could be perilous. IBM researchers wanted to make the fame machine-learning program Watson sound a little more human, so they introduced it to the web’s Urban Dictionary. While Watson learned some useful contemporary language from the site, it also picked up the website’s collection of vulgar slang and swear words. Unable to distinguish proper vocabulary from profanity, Watson developed a potty mouth and was banned from surfing the web unsupervised.
Algorithmic bias is becoming a major challenge at a critical moment in the evolution of machine learning and artificial intelligence. In her book Weapons of Math Destruction, Cathy O’Neil highlights the risk of algorithmic biases and people’s propensity to trust mathematical models because they believe they are objective and not swayed by human biases. The potential risks of automated systems that make vital business decisions based on uncontrolled data, and the willingness of humans to trust them blindly are promoting discussions about the need for algorithmic transparency and possibly regulating algorithms themselves.
Machine Learning and the Industrial Internet of Things
Applying self-learning systems in industrial settings is very appealing: Let learning algorithms analyze large repositories of equipment-generated data signatures and learn to detect abnormalities that might indicate an impending failure. Then, use data from past failure diagnoses and service actions to prescribe the most appropriate corrective action.
But like social data, machine data can be biased. What if the learned behavior is incorrect or is out of date? For instance, the training dataset may be from a class of machines that has since been modified. Or a corrective action that used to be a “best practice” is no longer recommended because it is obsolete, non-compliant, or is deemed unsafe.
Overall, the assumption that identical pieces of equipment exhibit similar behavior patterns that can be generalized is inaccurate. Especially mechanical devices, which are often the subject of machine learning monitoring schemes, are subject to mechanical and operational tolerances, wear and tear and other sources of operational variability that are always changing.
Teaching Learning Machines to Forget
The idea that a machine learning algorithm can learn continually by simply digesting torrents of equipment-generated data and provide an optimal course of action is both simplistic and optimistic. Machine learning systems cannot be built and left to their own devices. They need to be supervised. Every time there is a design change, a best practice is retired, or a failure mode is no longer happening, the old knowledge might need to be scrubbed.
With product design data and maintenance records scattered around the enterprise locked in functional silos and disparate datastores, the risk of information gaps, misinformation, and statistical biases is too high. Therefore, the knowledge lifecycle of artificial intelligence systems must be managed as an integral part of the product lifecycle, and should be updated in response to events such as design changes, a new supplier, or a new regulation.
The AI Pixie Dust Wears Off Quickly
Hopes and aspirations from machine learning systems are high. A 2017 PwC survey reports 72% of executives “believe AI will be the business advantage of the future.” And these corporate leaders demand action to capitalize on these new business opportunities.
But many of the hopeful do not understand the technology, and few technologists and AI software vendors have experience taking AI out of the controlled, PowerPoint-heavy, prototyping lab environment to the wild, where it must handle real-world scale and complexity challenges.
While progress in AI research and practical application will continue, companies should have realistic expectations about the effort to create reliable and scalable AI and machine learning systems. The effect of the AI pixie dust wears off quickly: building and validating AI is not a one-time effort. It can be a significant effort that requires continuous monitoring and updating throughout the product lifecycle.
Image: The Persistence of Memory(Salvador Dali, 1931)
The original article was edited July 2, 2019