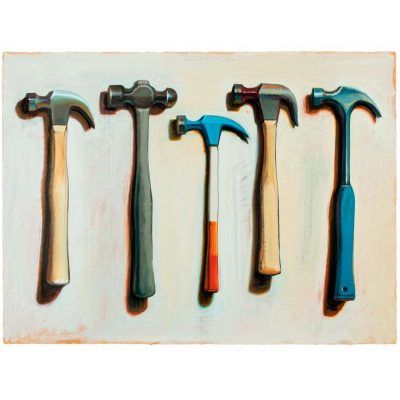
Everything is Artificial Intelligence
If your only tool you have is a hammer, then every problem looks like a nail. This overly used cliché seems very apropos when thinking about the rush to suggest artificial intelligence technology for practically every product and flaunt machine learning as a silver bullet for whatever problem the new product or service is purported to crack.
Indeed, AI technology can be very powerful. Recent advances in machine learning, aided by ubiquitous connectivity and distributed cloud computing, demonstrate how potent this technology is and promise much more to come. But AI-based systems can also be difficult to build and even more so to scale and deploy.
- Artificial intelligence systems are very impressive under limited controlled testing. But those with limited experienced with AI tend to underestimate the effort to generalize and validate prototypes for real-world situations.
- Certain types of machine learning algorithms are easily influenced by latent biases in the data used to train and validate them. Unbeknown to their users, these systems will repeat and amplify the same biases.
- The opaque nature of certain AI algorithms and software vendors that consider their algorithms proprietary and aren’t willing to discloses how they operate result in “unexplainable AI”: systems whose output cannot be easily validated and explained. Said differently, one cannot simply ask “why?” in order to examine the accuracy of the algorithm’s output. Errors, inaccuracies and biases are practically impossible to detect in advance and may be discovered only while the system is deployed.
AI-based systems are becoming increasingly powerful. But they can also be hard to validate, imperceptibly biased and arguably unethical. They threaten privacy and for some can be outright dangerous. No wonder the public opinion on AI is quite lukewarm. And similar concerns are often raised by AI scientists and in business circles.
But is artificial intelligence really the silver bullet it purports to be? Is it the only, or even the best technology to solve every problem and build every new product?
Or is AI becoming this proverbial hammer that we use indiscriminately to solve just about everything because it’s in vogue?
Are there other, more robust and proven approaches that have been around long enough and can accomplish the task at lower cost and risk? For instance, can clustering and regression analysis be used in place of a neural network to discover the relationships between data variables? Can statistical process control (SPC) and control charts provide the same level of asset monitoring and improve overall equipment effectiveness (OEE) as promised by machine learning systems, which are harder to calibrate? (In fact, some predictive maintenance systems touting machine learning are nothing more than old-fashioned SPC systems with fancy user interfaces…)
AI-based systems are rapidly becoming increasingly powerful. But they can also be imperceptibly biased, hard to validate and control, and arguably unethical, threaten privacy and outright dangerous. No wonder the public opinion on AI is quite lukewarm. And similar concerns are often raised by AI scientists and in business circles.
But is artificial intelligence really the silver bullet it purports to be? Is it the only, or even the best technology to solve every problem and build every new product?
Or is AI becoming this proverbial hammer that we use indiscriminately just because it’s in vogue?
Are there other robust and proven approaches that have been around long enough and can accomplish the task at lower cost and risk? For instance, can clustering and regression analysis be used in place of a neural network to discover the relationships between data variables? Can statistical process control (SPC) and control charts provide the same level of hardware monitoring and improve overall equipment effectiveness (OEE) as promised by machine learning systems? (In fact, some predictive maintenance systems touting machine learning are nothing more than old-fashioned SPC systems with fancy user interfaces…)
“When you are fundraising, it’s AI;
(Baron Schwartz)
When you are recruiting, it’s machine learning;
When you are implementing, it’s linear regression.”
Even when AI tools do offer superior classification, prediction and control policy planning capabilities, designers should consider using AI techniques for experimentation and prototyping, but use more robust methods and technologies for actual implementation, which can be validated and scaled faster and easier.
Choose your hammer prudently!