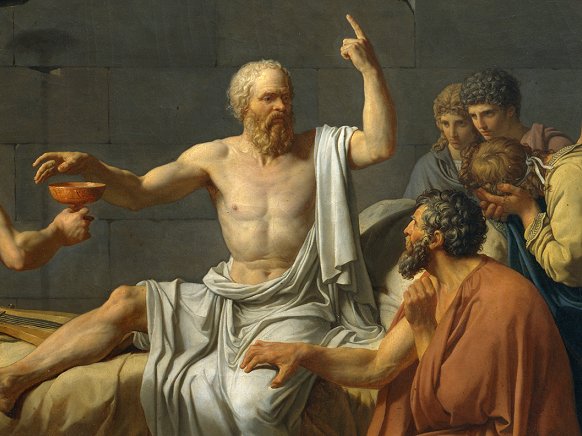
Artificial Intelligence and Machine Learning
Like the Internet of Things that shed its drab machine-to-machine image of a decade ago to become the cornerstone of the digital transformation movement, artificial intelligence technology is sprouting a new life from its 50-plus years old roots. (Yes, we’ve been doing artificial intelligence, admittedly with limited success, since the late 50s.)
There’s no doubt that AI has achieved impressive success over the last decade, promising a growing field of practical use of AI technology.
Most of the headline-grabbing narrative about AI revolves around deep leaning: development of neuron-like structures that “mimic the human brain” and learn by ingesting and analyzing massive amounts of data that contains patterns that exemplify the task the algorithm is to perform, such as word patterns for a voice recognition system. In other words, a machine learning algorithm is inductive: it uses data to generalize from instances to behavior, and responds to future inputs that are not necessarily identical to examples it has seen previously.
Like other AI methods, this concept of connectivism isn’t at all new. But advances in machine learning algorithms, aided by ubiquitous connectivity and distributed cloud computing make it possible to train neural nets on more data, and drastically improve performance.
Here Comes the Internet of Things
Many suggest that the Internet of Things (IoT) and the proliferation of IoT-connected devices will boost the deployment of smarter AI-based systems.
Early in the evolution of the IoT, analysts and pundits were obsessed with the ability to connect billions of IoT “things” to the Internet. Not only were most of these predictions proven overly optimistic, but the linkage between sheer connectivity and meaningful business outcomes was loose, at best.
We now witness a similar data deluge campaign: given enough data and clever computational techniques, we can make learning systems more “intelligent.”
But Is It Enough?
It’s true that it’s easier to solve problem that we think require “understanding” of complex context using a clever algorithm and lots of data by analyzing patterns and not trying to extract “meaning.”
Clever algorithms leveraging increased computational power of AI acceleration hardware and unprecedented amount of available digital information allow us to substitute the monumental task of representing complex knowledge into an optimization problem.
But the fixation on the ideas that more data is better for deep leaning is reminiscent of the obsession of counting IOT devices is finally replaced by focus on business outcomes enabled by the data generated by connected devices. The industry is gradually maturing from counting IoT conduits to measuring the value of their content.
In principle, the more data we harvest the smarter the AI system becomes.
But merely more IoT data and improved algorithms may do so much. Many, myself included, have raised concerns about the opaque nature of certain AI algorithms and about AI software vendors unwilling to discuss the design principles of their algorithms.
Opaque and “unexplainable” AI means we can build models, but we don’t know how they work. We can observe the system makes a recommendation, such selecting the best candidate from a pool of job applications, but we cannot ask “why?” and “how?” in order to examine the validity and accuracy of the algorithm. this type of systems can be imperceptibly biased and arguably unethical, characteristics that are practically impossible to detect in advance and may be discovered only while the system is in operation.
I’m Sorry Dave, I’m Afraid I Can’t Do That
The reader may argue that some degree of inability to explain complex decisions may be an innate and essential part of “intelligence.” This may be true. But at the same time, AI-based machines are our own creation. Will we trust and agree to cooperate with machines that are opaque and potentially irrefutable?
An article on the same topic and similar content was posted July 2018. This version has been edited substantially. You can read the original here.